Building a Multiple Criteria Decision Making model for PHOENIX with MACBETH
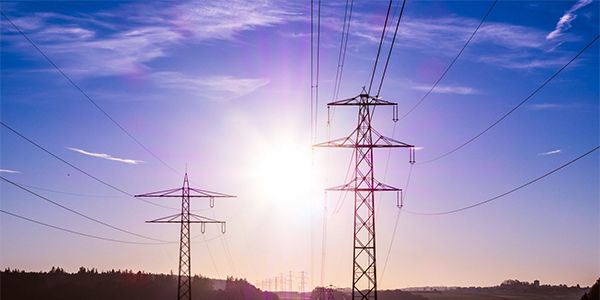
Evaluating the risk related to a cyberattack is very complex and must include many criteria. Taking into account the skills of subject matter experts is therefore capital. Multiple Criteria Decision Making is a very relevant tool to handle the complexity of the way the experts make their decision. One of the main issues in MCDA is the way we can collect their preferences and build the model. The MACBETH (Measuring attractiveness through a categorical-based evaluation technique) proposes an interesting methodology to deal with this issue in a scientific way.
Modeling the preferences of a Subject Matter Expert (SME) can be complicated. Several methods have been proposed to handle this complexity. In particular, MCDA models require a numerical calibration that allows SMEs to provide their preferences among the alternatives. Calibrating an MCDA model may seem simple but designing a method allowing the SMEs to provide a meaningful input is much more complicated than it looks. Indeed, a naive way of doing so would be to ask the SMEs to provide numerical inputs to the model. But experiments show that people are not good at making such row estimations. This is all the more so when the model is expressive and therefore requires more calibration. In PHOENIX, we rely on a very expressive MCDA model, based on the Choquet integral [1]. In our model, the number of parameters is possibly quadratic in the number of metrics; it may therefore be very complicated for the SMEs to provide numerical input for each of the parameters.
Hopefully, several methods have been designed to help designers interview the SMEs. Among them, the MACBETH [2] (Measuring attractiveness through a categorical-based evaluation technique) method allows to calibrate the model while only relying on qualitative input, which makes it much easier to handle for the SMEs.
The MACBETH method relies on the following principles: the SME is questioned over a series of alternatives and is asked to tell which is more attractive. They can provide three different kinds of feedback: an alternative is preferred to another one, the two alternatives are indifferent, or the SME cannot tell which is more attractive. For all the cases where the SME expresses a preference, they are also asked to provide the strength of this preference. As stated before, it is very hard for end users to provide numerical values, including for preferences. The SME can therefore pick a choice among a set of possible strength for their preference. Six levels are generally proposed: very weak; weak, moderate, strong, very strong and extreme. From there, it is possible to find out if the set of preferences provided by the SME is consistent or not and if so, to formalize the problem as a linear programming problem to find consistent values for the parameters of the model.
The MACBETH is generally applied to weighted sum models, but it has been proved to be applicable to more complex models, such as Choquet integrals [3]. This is how the MACBETH method is used in PHOENIX. The calibrated model is then used to define the risk level of a threat, and the relevance of mitigation, based on the features of the threats and mitigations.
[1] See How Multi-Criteria Decision Making can help choosing quickly accurate mitigation to a cyberattack and Fibre Tapping and New Advances in MCDA)
[2] See Bana e Costa, C. A., De Corte, J. M., & Vansnick, J. C. (2016). On the mathematical foundations of MACBETH. In Multiple criteria decision analysis (pp. 421-463). Springer, New York, NY.
[3] See Labreuche, C., & Grabisch, M. (2003). The Choquet integral for the aggregation of interval scales in multicriteria decision making. Fuzzy Sets and Systems, 137(1), 11-26. and Labreuche, C., Mayag, B., & Duqueroie, B. (2015). Extension of the MACBETH approach to elicit an ordered weighted average operator. EURO Journal on Decision Processes, 3(1), 65-105.