Why utilities adopting AI should put ethics first
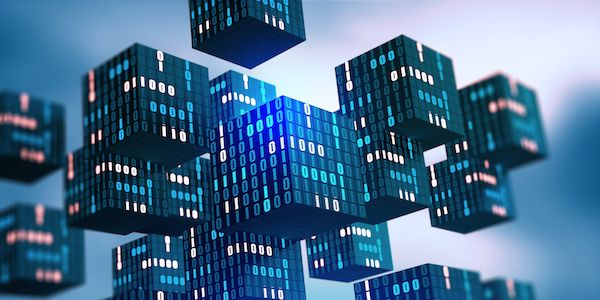
Artificial Intelligence (AI) is becoming vital for today’s increasingly complex utility business. Machine learning plays an integral part in the PHOENIX project, as well. In an earlier blog post, I discussed how digital technologies, such as AI, are essential to addressing the sustainability challenge[1]. Franck Freycenon recently published a blog exploring how AI can help energy and utility companies2.
Franck ended his post by noting that AI also brings with it new ‘digital dilemmas,’ the likes of which utilities have never seen before. In this blog post, I take a more in-depth look at these, specifically how bias could influence AI outcomes and why explainability is vital to ensuring humans maintain sufficient control. Let’s begin by looking at the question of bias.
Do AI algorithms contain bias?
Humans are not perfect. Humans have their own biases and cultural prejudices. And we can bring bias to AI algorithms – from the team that develops them and the data used to train them. I’m not talking about intentional bias, but bias simply because of human involvement in their creation.
So, we can expect AI algorithms to be imperfect too.
Let me give you an example. We developed an algorithm to explore customer data for energy theft detection. It flagged that affluent people were stealing energy. We initially thought there must be an error in the algorithm and considered discarding the findings, based purely on our cultural beliefs. When we checked later, affluent people were stealing energy. By rejecting those findings, we would have put our own cultural expectations into the algorithms, even if not intentionally. We would have influenced the algorithm’s training and future decision-making.
We must acknowledge that AI algorithms will contain bias.
How big an issue is bias?
Once we’re using AI, if it has bias built-in and is unable to do its job correctly, things could go very wrong. The impact could be immense:
- In power generation, bias could put lives at risk in safety-critical operations, or it could lead to costly mistakes in scenarios like energy trading where decisions need to be based on cost-effectiveness – whether to buy or produce electricity, for instance.
- On the customer engagement side, it could lead to negative customer experiences – if a customer is denied some services based on their profile or if we falsely accuse someone of fraud, for instance.
We must also remember the human side of energy when we use AI. When AI suggests products and services to offer individual customers, the bias in the data used by the AI algorithm may lead to unintentional discrimination. The consequences could have a dramatic impact on people’s lives, particularly concerning energy poverty.
Let me give two examples, one in the developing world and the other in the developed world:
- In developing countries, electrification is elevating society’s capabilities. We need to ensure AI’s decisions don’t cut off electricity access, which would prevent children from studying after dark and get in the way of their education.
- In developed countries, utilities must provide citizens’ basics energy needs. We need to ensure AI looks beyond the maximizing sales perspective. Wrong decisions could drag population groups already in fuel poverty even deeper into debt and raise social inequality.
We must acknowledge that the outcomes of AI algorithms could lead to social discrimination as well as financial and safety consequences. As we just discussed, we need to ensure we address these dimensions in our design of business services based on AI algorithms.
Can we trust AI to make the right decisions?
We sometimes find the outcome of an algorithm can be quite surprising, even creative in the sense that no human would have come to the same conclusion – which is most often a good thing.
But when offering customers services based on data where bias may exist in the data, we need to know why the AI is suggesting specific outcomes and not others. And this is where we come to explainability.
Most AI algorithms are essentially black box pattern detectors. They can detect specific patterns quickly, but they don’t give you the causality of why those patterns happen. We can’t see why an algorithm has taken a decision; it’s not explained in a way that can be understood by a human.
And we are making decisions based on these ‘black boxes’ without knowing why. We are losing control over the way decisions are being made – and this could be an issue.
When we are using AI for dealing with customers, we must avoid unjustified discrimination. But when we are using AI for infrastructure – for managing the grid or for generation, for example – taking operational decisions purely on the outcomes of these opaque algorithms can bring risks because they could sometimes fail without us knowing why. With extreme levels of automation, we could encounter safety issues.
There clearly needs to be some level of human control over what is happening. And research in the field of explainability aims to do just that. Researchers are studying how black box algorithms can provide humans with different mechanisms for understanding the decisions they may make.
Ethics will be crucial to the success of systems based on AI. The challenges utility companies face around ethics will only grow as machines advance, and their adoption grows.
[1] https://atos.net/en/blog/why-digitalizing-the-energy-value-chain-is-fundamental-to-saving-our-planet