Federated Learning for enhancing the security of Critical Energy Infrastructures
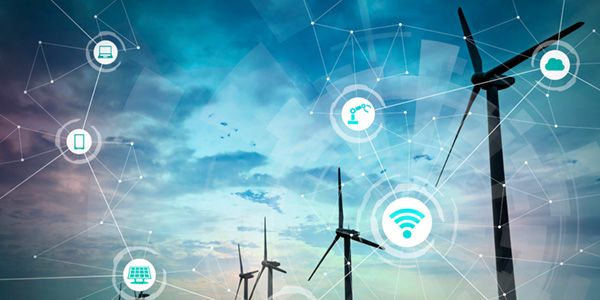
Wind-turbine systems are considered among the most complex Cyber-Physical infrastructures causing huge cascading effects to other Critical Energy Infrastructures (CEIs), such as Electrical Power and Energy Systems (EPES), communications, transportation, industry and finance. Wind turbines are mainly composed of condition monitoring and operational data (i.e. Supervisory Command and Data Acquisition-SCADA), including air-temperature, air-pressure, voltage and power with multiple types of parameters and periodic characteristics. Compared with legacy SCADA systems, recent-developed infrastructures utilize less expensive and more scalable Internet-based technologies that enable data monitoring in near real-time conditions.
However, the main limitations regarding wind turbine data monitoring still pertain. An innovative approach is to adopt Privacy-Preserving Federated Machine Learning solutions in order to detect any possible anomalies in such infrastructures. Instead of centralizing the wind-turbine data into a common server, Federated Machine Learning allows the data to remain on-premise in the infrastructure. This enables the responsible authorities to consider the advantages of Machine Learning, and simultaneously protect their privacy. Federated learning is able to train a model using data stored at multiple wind-turbine stations without the data leaving the station’s premises, as it is illustrated in Figure 1.
This procedure is implemented by first training separate models at each wind-turbine station where the local data is available, and then by distributing these models to a central server that combines them into a global model. When the wind-turbine station acquires more SCADA measurements, it can download the latest global model from the server, update it with the new data, and send it back to the server. The specific process guarantees that raw data is never exchanged—only their local models.
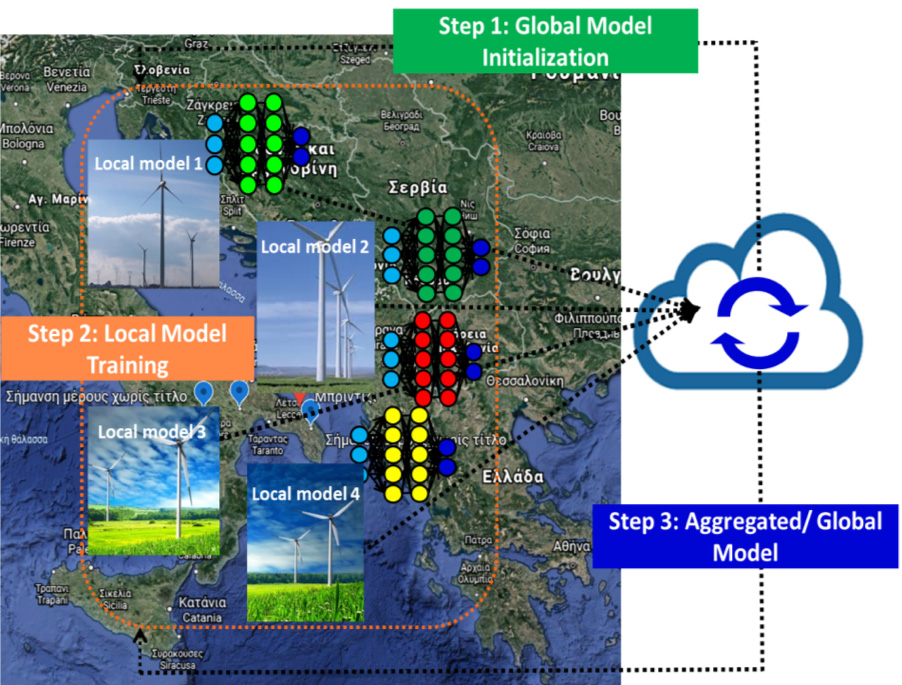
Within the PHOENIX project, we design and develop such innovative formulations that enhance the privacy of Critical Energy Infrastructures.